Python Binomial Distribution With Scipy Library

Scipy Python Library Studyopedia Scipy.stats.binom# scipy.stats. binom = <scipy.stats. discrete distns.binom gen object> [source] # a binomial discrete random variable. as an instance of the rv discrete class, binom object inherits from it a collection of generic methods (see below for the full list), and completes them with details specific for this particular distribution. Binomial distribution. #. a binomial random variable with parameters (n, p) can be described as the sum of n independent bernoulli random variables of parameter p; y = ∑ i = 1 n x i. therefore, this random variable counts the number of successes in n independent trials of a random experiment where the probability of success is p.
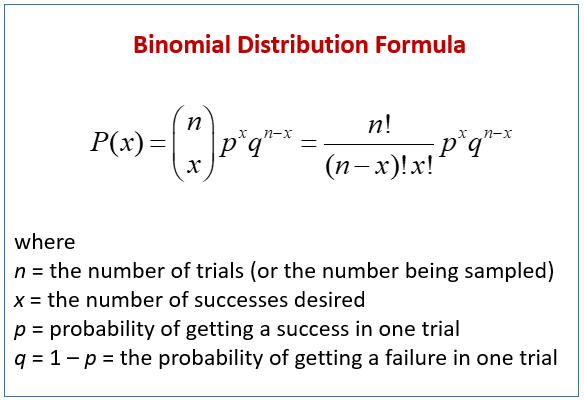
Binomial Distribution In Python Delft Stack Parameters n: number of successes n: sample size pct: the size of the confidence interval (between 0 and 1) a: the alpha hyper parameter for the beta distribution used as a prior (default=1) b: the beta hyper parameter for the beta distribution used as a prior (default=1) n pbins: the number of bins to segment the p range into (default. Instructional video on creating a probability mass function and cumulative density function of the binomial distribution in python using the scipy library.co. We will do this by using the binomial distribution: it means the following: p (x = k) — the probability of obtaining k successful outcomes in a total of n independent trials. n — the number of trials. (in this case, 21.) k — the number of successes. (in this case, heads.) p — the chance that a trial is successful. Statistical functions (. scipy.stats. ) #. this module contains a large number of probability distributions, summary and frequency statistics, correlation functions and statistical tests, masked statistics, kernel density estimation, quasi monte carlo functionality, and more. statistics is a very large area, and there are topics that are out of.

Scipy Stats Complete Guide Python Guides 2022 We will do this by using the binomial distribution: it means the following: p (x = k) — the probability of obtaining k successful outcomes in a total of n independent trials. n — the number of trials. (in this case, 21.) k — the number of successes. (in this case, heads.) p — the chance that a trial is successful. Statistical functions (. scipy.stats. ) #. this module contains a large number of probability distributions, summary and frequency statistics, correlation functions and statistical tests, masked statistics, kernel density estimation, quasi monte carlo functionality, and more. statistics is a very large area, and there are topics that are out of. You can visualize a binomial distribution in python by using the seaborn and matplotlib libraries: from numpy import random. import matplotlib.pyplot as plt. import seaborn as sns. x = random.binomial(n=10, p=0.5, size=1000) sns.distplot(x, hist=true, kde=false) plt.show() the x axis describes the number of successes during 10 trials and the y. To explore the bernoulli distribution in python, we will be using a hypothetical lottery ticket with a 10% chance of winning: #import scipy.stats library from scipy.stats import bernoulli. #the lottery ticket is a bernoulli random variable with p=0.1. lottery = bernoulli(p=0.1) setting up our bernoulli random variable: lottery.
Comments are closed.