How To Effectively Use The Data Science Lifecycle
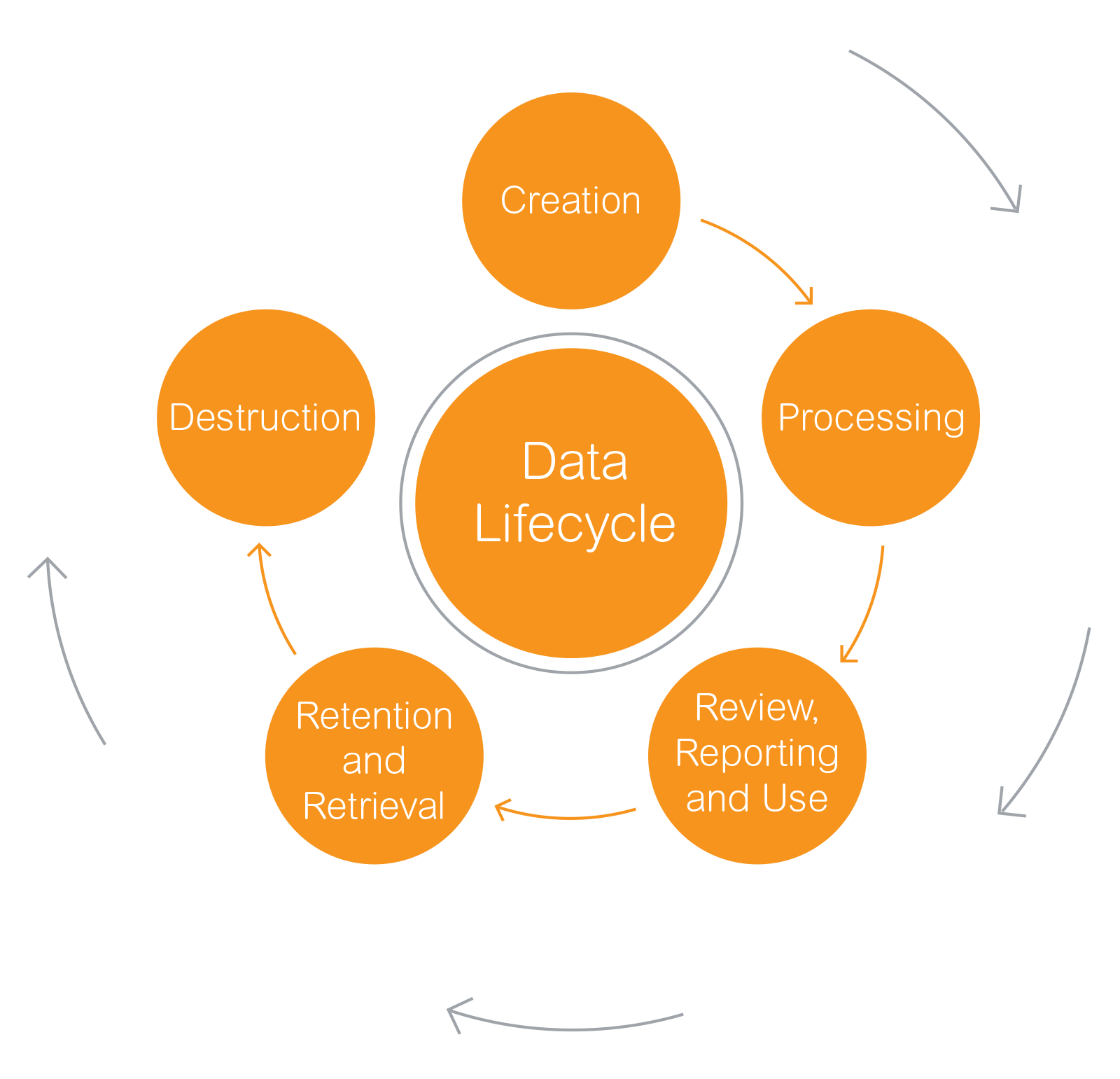
Data Lifecycle Diagram It is, therefore, important to know the data science lifecycle when applying data to extract information and solve problems. this lifecycle encompasses five key stages, that include—data collection, data pre processing, data processing, data mining, and data presentation and dissemination. all of these are crucial in the process of converting. In this exploration of the data science lifecycle, we've covered the various stages that data scientists and analysts follow to effectively harness data and create valuable insights. let's recap the key stages and discuss the importance of a systematic approach in data science, as well as some future trends and evolutions in data science.
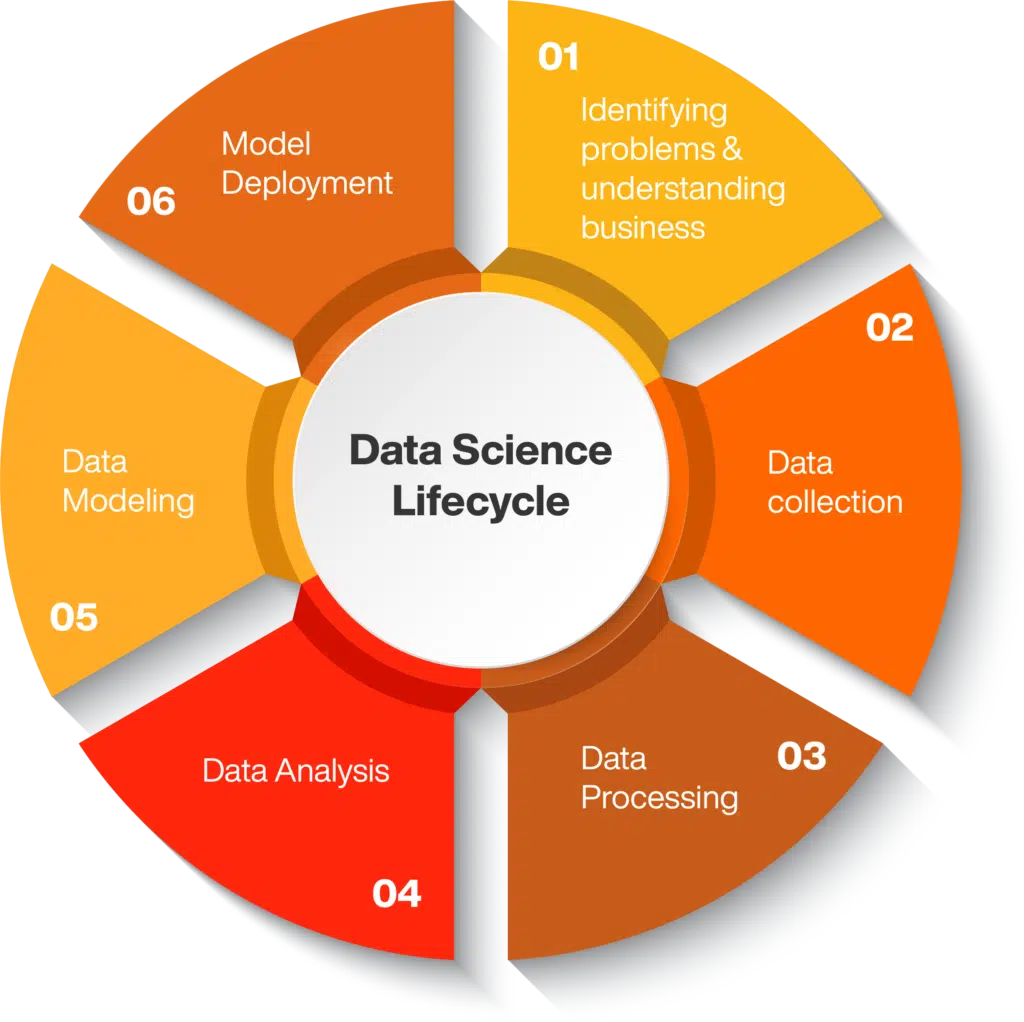
What Is The Data Science Lifecycle Online Manipal The data science lifecycle is a framework that provides a structured approach to solving real world problems using data. in this blog post, we will explore the different stages of the data science lifecycle and how to implement them effectively. It provides a framework for data scientists to follow from problem definition to model evaluation. the data science lifecycle encompasses five main stages, each with its own set of tasks and goals. these stages are: defining the problem. data collection and preparation. data exploration and analysis. model building and evaluation. The data science lifecycle is a framework that guides data science projects from conception to deployment. understanding each phase ensures that projects are executed efficiently and effectively. The data science life cycle is a crucial process that helps to ensure accurate and effective models are produced. by following the six key steps of problem definition, data collection and exploration, data cleaning and preprocessing, data analysis and modeling, evaluation, and deployment, data scientists can ensure that their models are robust.

12 Typical Data Science Lifecycle Download Scientific Diagram The data science lifecycle is a framework that guides data science projects from conception to deployment. understanding each phase ensures that projects are executed efficiently and effectively. The data science life cycle is a crucial process that helps to ensure accurate and effective models are produced. by following the six key steps of problem definition, data collection and exploration, data cleaning and preprocessing, data analysis and modeling, evaluation, and deployment, data scientists can ensure that their models are robust. Step 9 monitoring and maintenance. the data science lifecycle doesn't end with deployment; continuous monitoring is essential to ensure the model performs well over time. monitoring involves tracking model accuracy, detecting drift in data patterns, and updating the model as needed. The data science life cycle is simply the series of steps a data scientist—or another related professional—takes to complete the process of solving a problem for an organisation using large amounts of data and various other tools. everyone's data science life cycle may look slightly different, but they all include the same six basic steps.
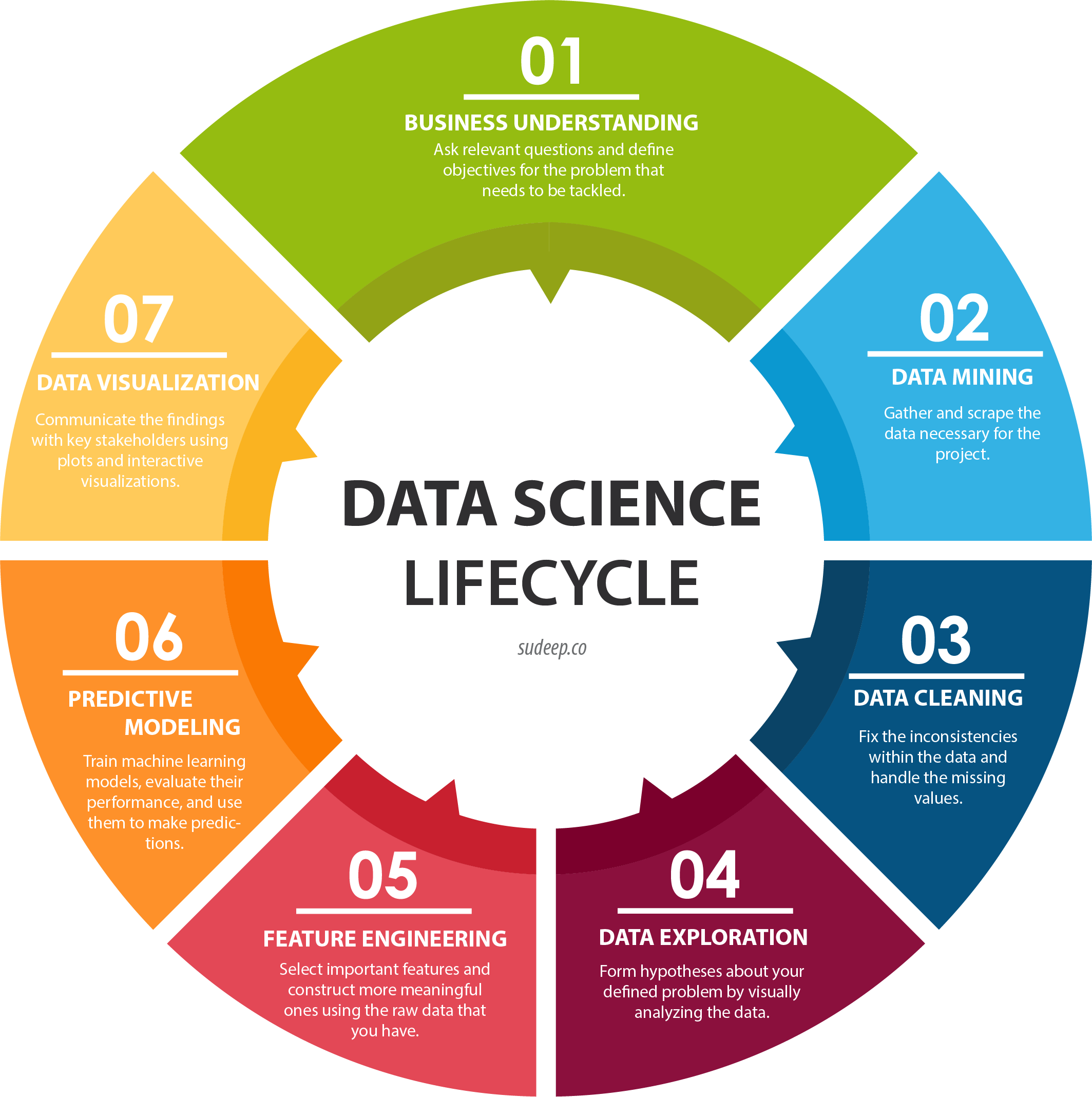
Understanding The Data Science Lifecycle Step 9 monitoring and maintenance. the data science lifecycle doesn't end with deployment; continuous monitoring is essential to ensure the model performs well over time. monitoring involves tracking model accuracy, detecting drift in data patterns, and updating the model as needed. The data science life cycle is simply the series of steps a data scientist—or another related professional—takes to complete the process of solving a problem for an organisation using large amounts of data and various other tools. everyone's data science life cycle may look slightly different, but they all include the same six basic steps.
Comments are closed.