Hierarchical Reinforcement Learning On Robots With Options
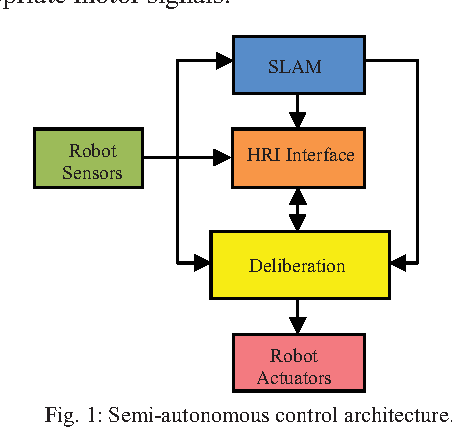
Figure 1 From A Hierarchical Reinforcement Learning Based Control It can also reduce the detrimental effects of redundant continuous options on policy learning. however, too frequent switching is not conducive to stable performance and may even result in a hierarchical degradation dilemma. thus, we propose the hierarchical reinforcement learning with adaptive scheduling (has) algorithm to address these issues. The hierarchical reinforcement learning with the unlimited option scheduling (uos) algorithm is proposed. unlike conventional o hrl algorithms that apply a limited set of options with specific meanings, uos encourages an infinite number of options to correlate with trajectories while maintaining a correlation with each other, thus representing.
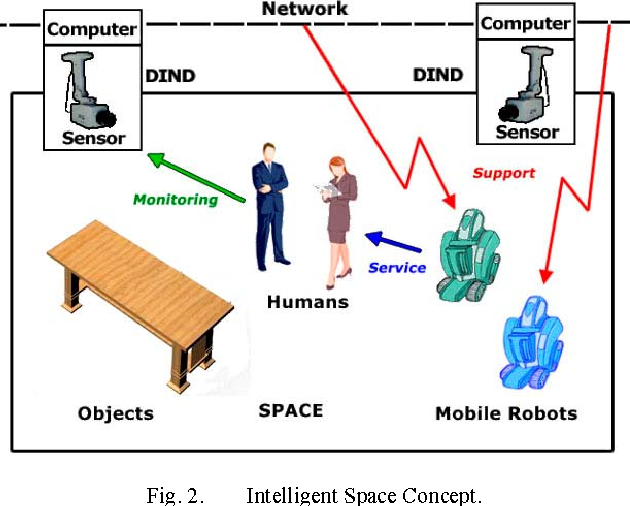
Figure 1 From Hierarchical Reinforcement Learning For Robot Navigation Hierarchical reinforcement learning is a promising computational approach that may eventually yield comparable problem solving behaviour in artificial agents and robots. To design an available scheduling method for continuous options, in this paper, the hierarchical reinforcement learning with adaptive scheduling (has) algorithm is proposed. its low level controller learns diverse options, while the high level controller schedules options to learn solutions. it achieves an adaptive balance between exploration. We propose a hierarchical reinforcement learning method, hidio, that can learn task agnostic options in a self supervised manner while jointly learning to utilize them to solve sparse reward tasks. unlike current hierarchical rl approaches that tend to formulate goal reaching low level tasks or pre define ad hoc lower level policies, hidio encourages lower level option learning that is. To overcome the aforementioned limitations, learning approaches—such as hierarchical reinforcement learning 13 —is an alternative approach to accomplish tasks that require solving a discrete.
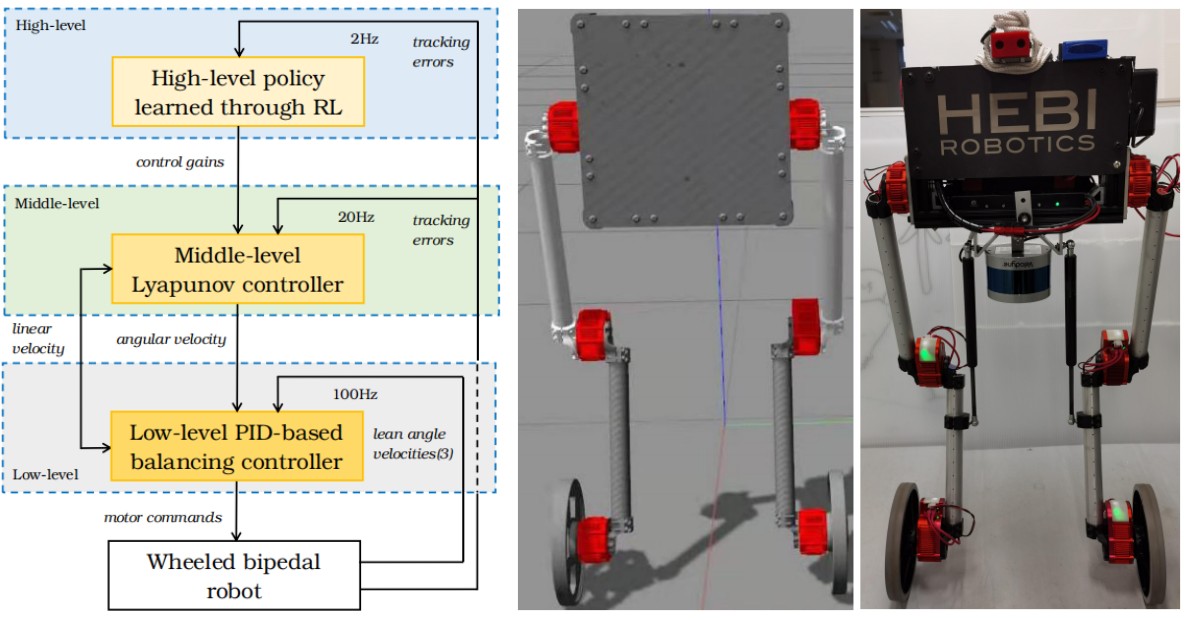
Reinforcement Learning Based Hierarchical Control For Path Tracking Of We propose a hierarchical reinforcement learning method, hidio, that can learn task agnostic options in a self supervised manner while jointly learning to utilize them to solve sparse reward tasks. unlike current hierarchical rl approaches that tend to formulate goal reaching low level tasks or pre define ad hoc lower level policies, hidio encourages lower level option learning that is. To overcome the aforementioned limitations, learning approaches—such as hierarchical reinforcement learning 13 —is an alternative approach to accomplish tasks that require solving a discrete. Our approach defines options in hierarchical reinforcement learning (hrl) from aip operators by establishing a corre spondence between the state transition model of ai planning problem and the abstract state transition system of a markov decision process (mdp). options are learned by adding in trinsic rewards to encourage consistency between. Recent literature in the robot learning community has focused on learning robot skills that abstract out lower level details of robot control, such as dynamic movement primitives (dmps) ijspeert et al. [2013], the options framework in hierarchical rl, and subtask policies [leslie pack kaelbling, 2017, andreas et al., 2016, neumann et al., 2014].

Hierarchical Reinforcement Learning On Robots With Options Youtube Our approach defines options in hierarchical reinforcement learning (hrl) from aip operators by establishing a corre spondence between the state transition model of ai planning problem and the abstract state transition system of a markov decision process (mdp). options are learned by adding in trinsic rewards to encourage consistency between. Recent literature in the robot learning community has focused on learning robot skills that abstract out lower level details of robot control, such as dynamic movement primitives (dmps) ijspeert et al. [2013], the options framework in hierarchical rl, and subtask policies [leslie pack kaelbling, 2017, andreas et al., 2016, neumann et al., 2014].
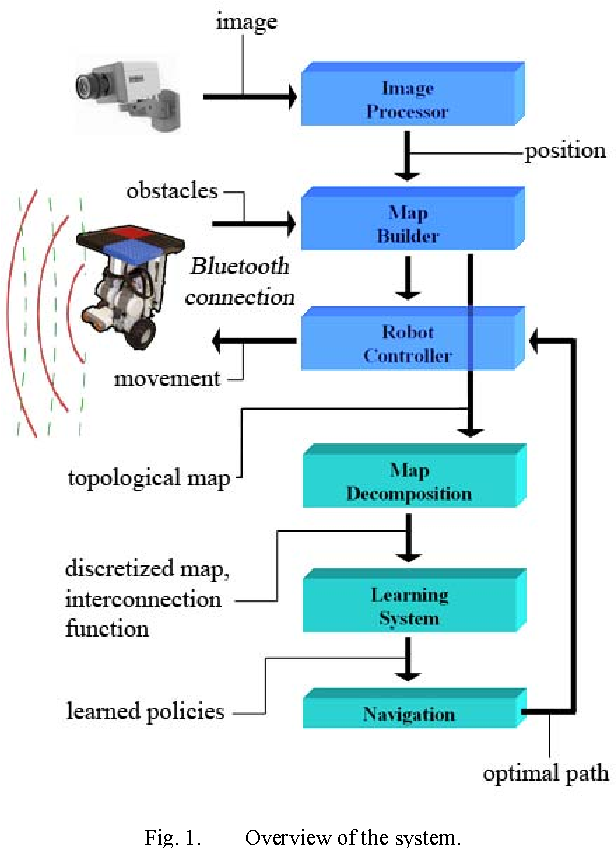
Figure 1 From Hierarchical Reinforcement Learning For Robot Navigation
Comments are closed.