Frontiers Accelerated Atomistic Modeling Of Solid State Battery
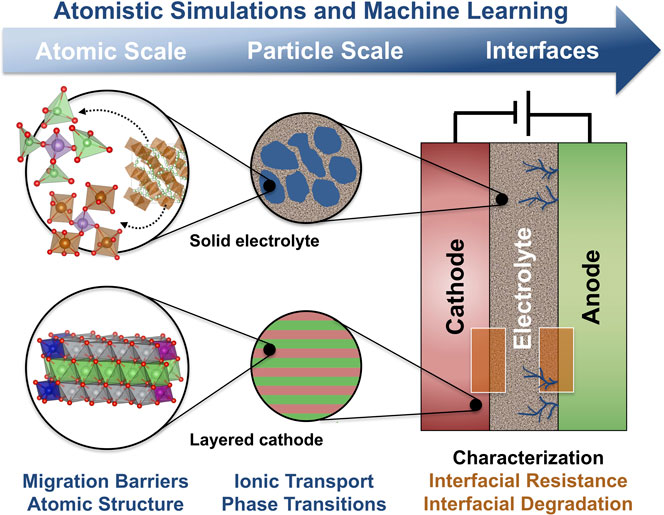
Frontiers Accelerated Atomistic Modeling Of Solid State Battery Figure 1. the properties of solid state batteries, such as stability and rate capability, are often dependent on atomic scale phenomena, such as phase changes or structural motifs at interfaces and grain boundaries. machine learning techniques can increase the length and time scale that atomistic simulations can access. Frontiers in energy research received: 15 april 2021 accepted: 18 may 2021 published: 04 june 2021 citation: guo h,wangq, stukea, urbanaand artrith n (2021) accelerated atomistic modeling of solid state battery materials with machine learning. front. energy res. 9:695902. doi: 10.3389 fenrg.2021.695902.
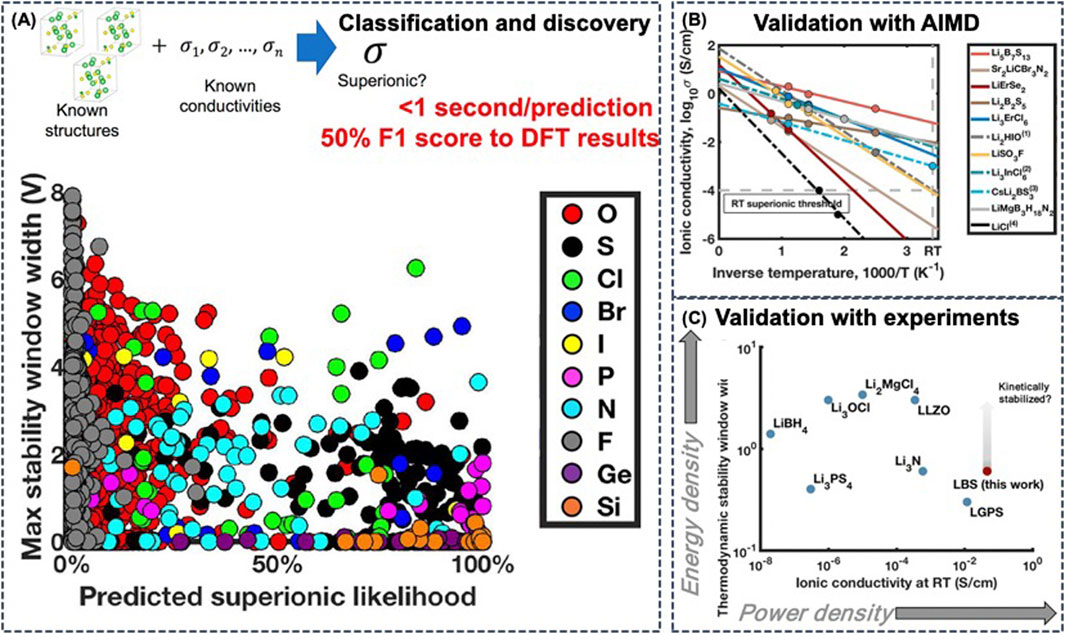
Frontiers Accelerated Atomistic Modeling Of Solid State Battery Abstract and figures. materials for solid state batteries often exhibit complex chemical compositions, defects, and disorder, making both experimental characterization and direct modeling with. Doi: 10.3389 fenrg.2021.695902 corpus id: 235326097; accelerated atomistic modeling of solid state battery materials with machine learning @inproceedings{guo2021acceleratedam, title={accelerated atomistic modeling of solid state battery materials with machine learning}, author={haoyue guo and qian wang and annika stuke and alexander urban and nongnuch artrith}, booktitle={frontiers in energy. <jats:p>materials for solid state batteries often exhibit complex chemical compositions, defects, and disorder, making both experimental characterizat. Here, we give an overview of recent methodological advancements of ml techniques for atomic scale modeling and materials design. we review applications to materials for solid state batteries, including electrodes, solid electrolytes, coatings, and the complex interfaces involved. solid state batteries. interfaces. atomistic simulations.
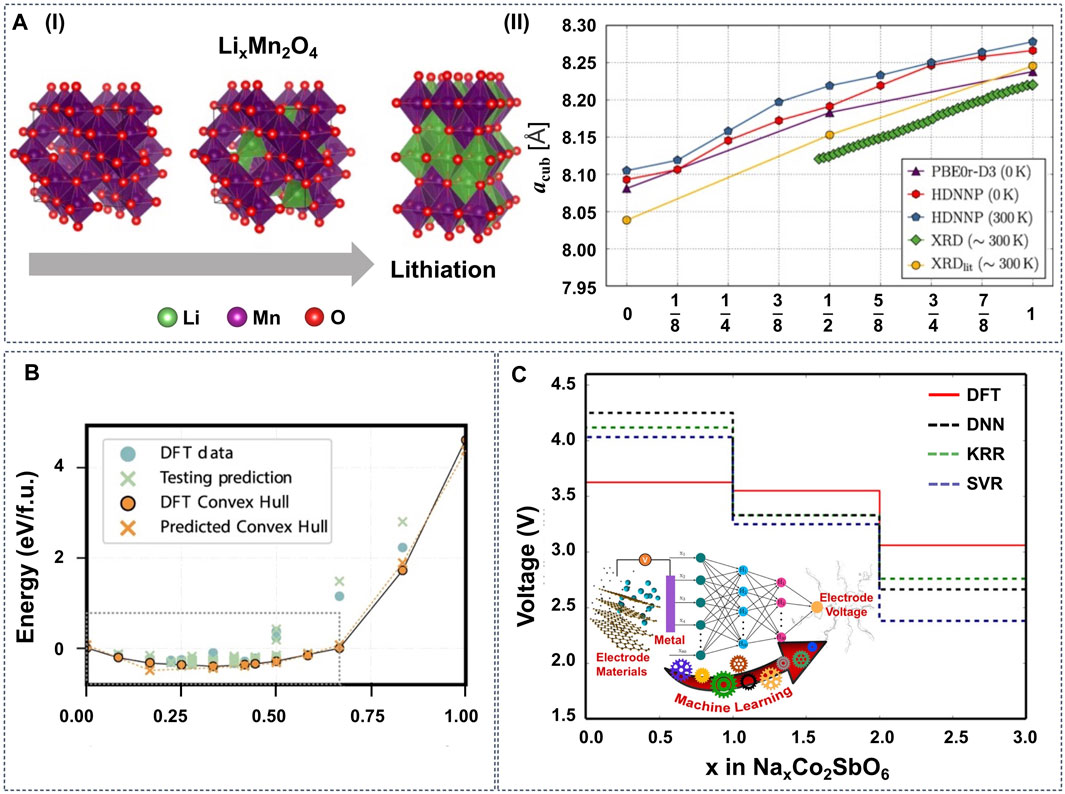
Frontiers Accelerated Atomistic Modeling Of Solid State Battery <jats:p>materials for solid state batteries often exhibit complex chemical compositions, defects, and disorder, making both experimental characterizat. Here, we give an overview of recent methodological advancements of ml techniques for atomic scale modeling and materials design. we review applications to materials for solid state batteries, including electrodes, solid electrolytes, coatings, and the complex interfaces involved. solid state batteries. interfaces. atomistic simulations. We review applications to materials for solid state batteries, including electrodes, solid electrolytes, coatings, and the complex interfaces involved. report this publication. accelerated atomistic modeling of solid state battery materials with machine learning: materials for solid state batteries often exhibit complex chemical compos. The increasing demand for substantial and efficient energy storage system has driven considerable research into advanced battery materials, such as lithium air, sodium air, lithium sulfur, and solid electrolyte batteries. these materials offer promising solutions for improved energy density and safety in next generation batteries. however, their complex physical and chemical properties have.
Comments are closed.