Figure 1 From Single And Multi Objective Evolutionary Algorithms For
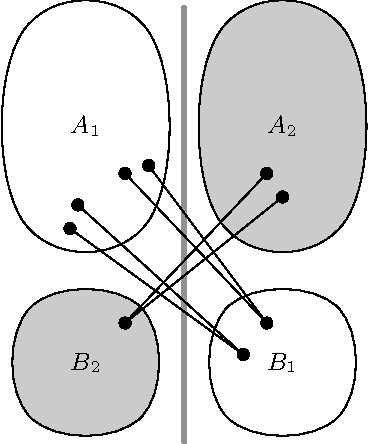
Figure 1 From Single And Multi Objective Evolutionary Algorithms For Abstract. several reviews have been made regarding the methods and application of multi objective optimization (moo). there are two methods of moo that do not require complicated mathematical equations, so the problem becomes simple. these two methods are the pareto and scalarization. in the pareto method, there is a dominated solution and a. Portrays a trade o among objectives, in a single simulation run. in this chapter, we present a brief description of an evolutionary optimization procedure for single objective optimization. thereafter, we describe the principles of evolutionary multi objective optimization. then, we discuss some salient developments in emo research.
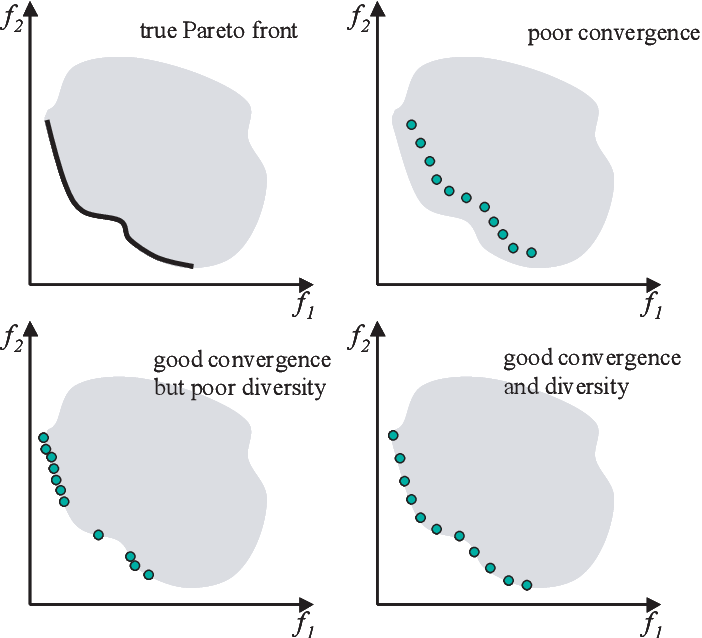
Figure 1 From Multi Objective Evolutionary Algorithms Semantic S 1 altmetric. metrics. simultaneous optimization of several competing objectives requires increasing the capability of optimization algorithms. this paper proposes the multi objective moth swarm. Archive based single objective evolutionary algorithms for submodular optimization a preprint figure 1: gray set ain the left figure is a subset of the gray setbin the middle figure which in turn is a subset of the gray set cin the right figure. adding the blue set leads to a lower gain of area the larger the gray set is. Abstract. pareto based multi objective evolutionary algorithms experience grand challenges in solving many objective optimization problems due to their inability to maintain both convergence and diversity in a high dimensional objective space. exiting approaches usually modify the selection criteria to overcome this issue. different from them, we propose a novel meta objective (meo) approach. Community detection is a crucial research direction in the analysis of complex networks and has been shown to be an np hard problem (a problem that is at least as hard as the hardest problems in nondeterministic polynomial time). multi objective evolutionary algorithms (moeas) have demonstrated promising performance in community detection. given that distinct crossover operators are suitable.
Comments are closed.