Figure 1 From Evolutionary Multi Tasking Single Objective Optimization
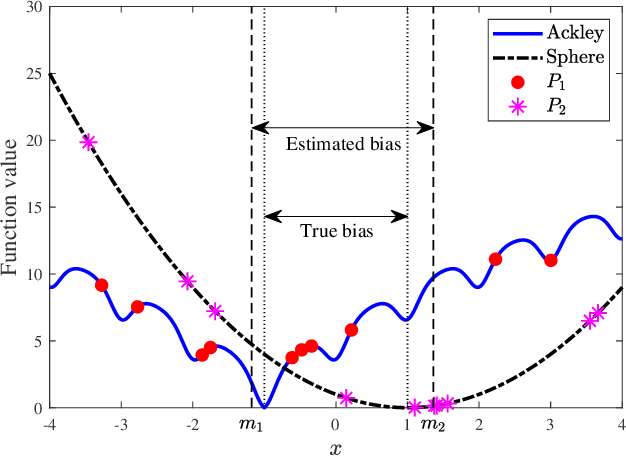
Figure 1 From Multi Tasking Evolutionary Algorithm Mtea For Singleо Herein, evolutionary multitasking enables autonomous transfer of knowledge from one problem to the next, thereby naturally augmenting the effectiveness of the search. in addition to enhancing productivity, the process of multi tasking can often provide superior quality solutions in compar ison to traditional single task optimization. this is. An adaptive evolutionary multi task optimization algorithm that uses a multi population evolutionary framework to solve multiple tasks in parallel, allocating different computational resources to different tasks and dynamically adjusts the amount of knowledge transfer according to the survival rate of offspring and transferred individuals is proposed.
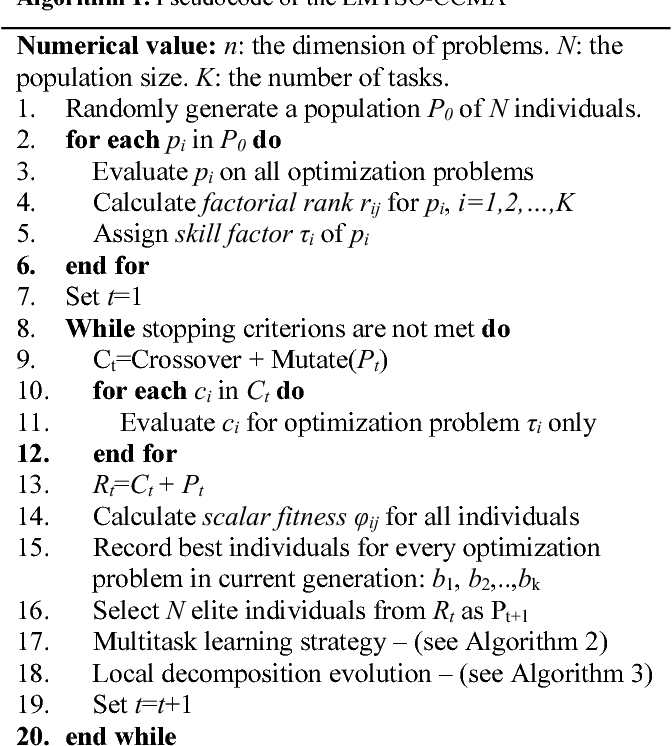
Figure 1 From Evolutionary Multi Tasking Single Objective Optimization Evolutionary multi tasking optimization has recently emerged as a promising new topic in the field of evolutionary computation. it is a promising framework for solving different optimization problems simultaneously. compared with the classic evolutionary algorithms, evolutionary multi tasking optimization (mto) can take advantage of implicit genetic transfer in the optimization process and get. The first test suite cec2019 somtp [36] is the single objective multitasking standard test set, which comprises nine single objective mto problems, each consisting of two minimization tasks. 1. introduction. evolutionary algorithms (eas) [1] have a better ability of global search by simulating the process of natural evolution, which have been used to solve many complex optimization problems, such as single objective optimization problems (sops) [2], multi objective optimization problems (mops) [3], multi modal optimization problems (mmops) [4], and dynamic optimization problems. The same optimization task, in contrast, evolutionary multi tasking tackles multiple distinct optimization tasks at once. as shown in fig 1, f 1;f 2;:::;f k represent the objective functions of each task separately. the aim of evolutionary multitasking is to get the best solution for each of the.
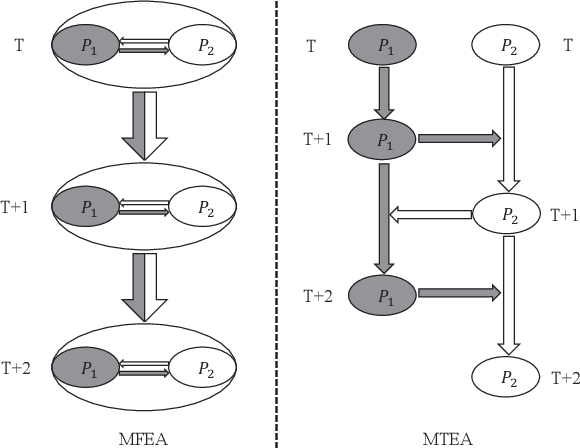
Figure 1 From Multi Tasking Evolutionary Algorithm Mtea For Singleо 1. introduction. evolutionary algorithms (eas) [1] have a better ability of global search by simulating the process of natural evolution, which have been used to solve many complex optimization problems, such as single objective optimization problems (sops) [2], multi objective optimization problems (mops) [3], multi modal optimization problems (mmops) [4], and dynamic optimization problems. The same optimization task, in contrast, evolutionary multi tasking tackles multiple distinct optimization tasks at once. as shown in fig 1, f 1;f 2;:::;f k represent the objective functions of each task separately. the aim of evolutionary multitasking is to get the best solution for each of the. Many existing methods transfer elite solutions between tasks to improve algorithm performance, however, these methods may or produce negative transfer if inter task similarity is low or irrelevant. this paper presents a self adaptive multitasking optimization algorithm, samtoa, to find more valuable transferred solutions between tasks. Presents the first comprehensive and systematic introduction to evolutionary multi task (emt) optimization. describes in detail the application of emt algorithms in solving various optimization problems. written by leading experts in the field of evolutionary computation. part of the book series: machine learning: foundations, methodologies.
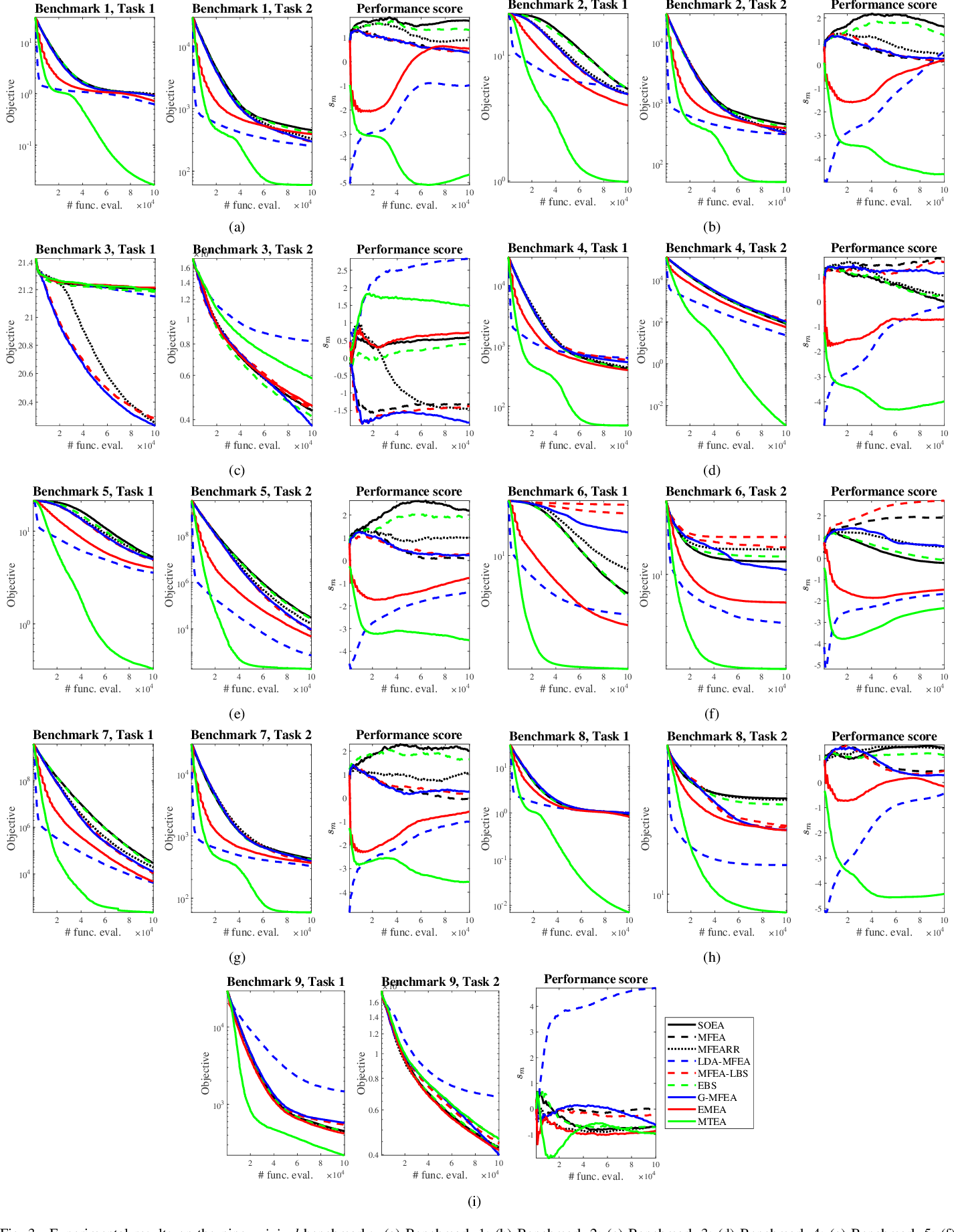
Figure 1 From Multi Tasking Evolutionary Algorithm Mtea For Singleо Many existing methods transfer elite solutions between tasks to improve algorithm performance, however, these methods may or produce negative transfer if inter task similarity is low or irrelevant. this paper presents a self adaptive multitasking optimization algorithm, samtoa, to find more valuable transferred solutions between tasks. Presents the first comprehensive and systematic introduction to evolutionary multi task (emt) optimization. describes in detail the application of emt algorithms in solving various optimization problems. written by leading experts in the field of evolutionary computation. part of the book series: machine learning: foundations, methodologies.
Comments are closed.