Expectation Of Linear Combination Youtube

Expectation Of Linear Combination Youtube An introduction to the rules of expectation and variance when we have a linear combination of independent variables. Statsresource.github.io random variables linear combinations of random variablesstatistics and probability tutorial videos worked examples and demonstr.

Intermediate Sme Lecture 4 Central Limit Theorem Expectation We look at some theroems that may provide some time saving techniques for computing expectations.the content in this course follows along with the 9th editio. Combining properties of linear rescaling with linearity of expected value yields the expected value of a linear combination. e (a x b y) = a e (x) b e (y) linearity of expected value extends naturally to more than two random variables. example 24.2. recall the matching problem in example 21.1. A linear rescaling is a transformation of the form \(g(u) = au b\). recall that in section 4.6.1 we observed, via simulation, that. a linear rescaling of a random variable does not change the basic shape of its distribution, just the range of possible values. a linear rescaling transforms the mean in the same way the individual values are. Linear combinations of random variables. expected value of a constant. expectation of a product of random variables. non linear transformations. addition of a constant matrix and a matrix with random entries. multiplication of a constant matrix and a matrix with random entries. expectation of a positive random variable. preservation of almost.

Calculating The Expectation And Variance For A Linear Combination Of X A linear rescaling is a transformation of the form \(g(u) = au b\). recall that in section 4.6.1 we observed, via simulation, that. a linear rescaling of a random variable does not change the basic shape of its distribution, just the range of possible values. a linear rescaling transforms the mean in the same way the individual values are. Linear combinations of random variables. expected value of a constant. expectation of a product of random variables. non linear transformations. addition of a constant matrix and a matrix with random entries. multiplication of a constant matrix and a matrix with random entries. expectation of a positive random variable. preservation of almost. I have already written out the expectation of y out following properties of expectation: ey = aex1 bex2 e y = a e x 1 b e x 2. and to obtain ex1 e x 1 i can write ey−bex2 a e y − b e x 2 a; however, how would i get rid of the ex2 e x 2? essentially, i can write out the expectations of linear combinations, but i have no idea how to write. 2. i am studying conditional expectations and am stuck with this issue. consider an expectation conditional on a fixed sum of random variables, e.g. e[x| x y = α] e [x | x y = α]. then we can retrieve it from joint density f(x, y) f (x, y) as in here. what if our conditional part is more complicated such as a linear combination of random.
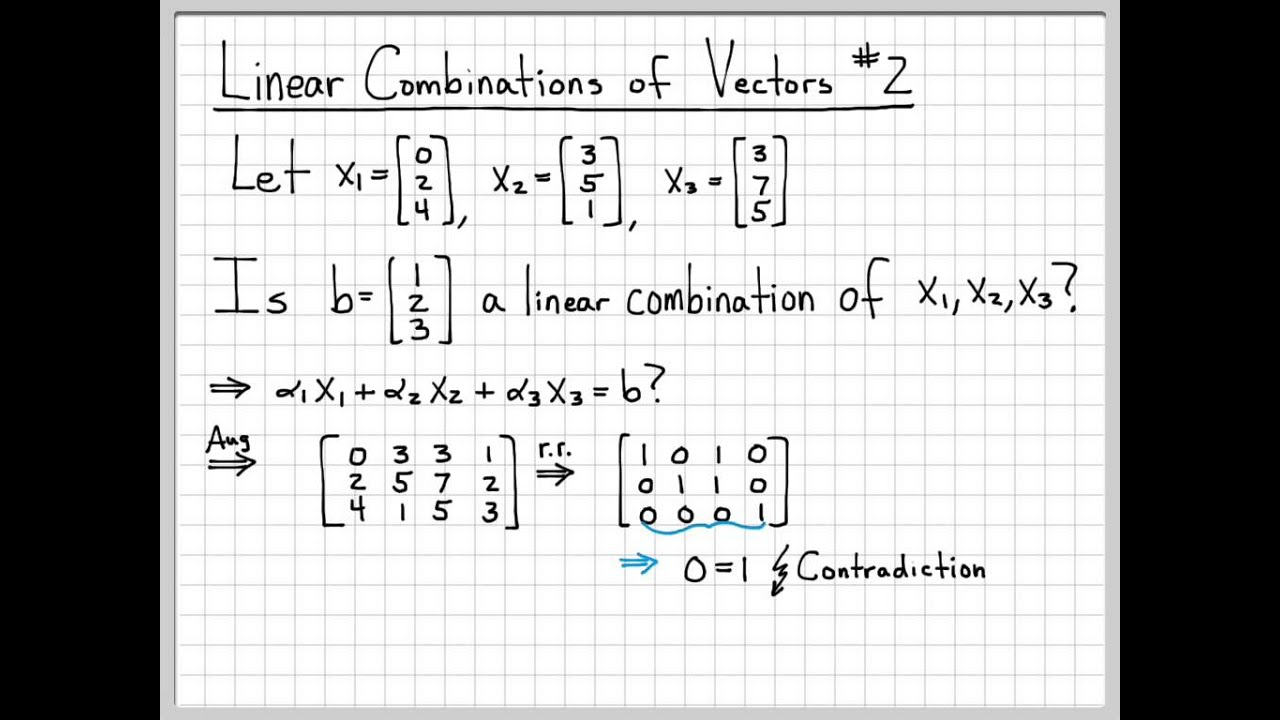
Linear Algebra Example Problems Linear Combination Of Vectors 2 I have already written out the expectation of y out following properties of expectation: ey = aex1 bex2 e y = a e x 1 b e x 2. and to obtain ex1 e x 1 i can write ey−bex2 a e y − b e x 2 a; however, how would i get rid of the ex2 e x 2? essentially, i can write out the expectations of linear combinations, but i have no idea how to write. 2. i am studying conditional expectations and am stuck with this issue. consider an expectation conditional on a fixed sum of random variables, e.g. e[x| x y = α] e [x | x y = α]. then we can retrieve it from joint density f(x, y) f (x, y) as in here. what if our conditional part is more complicated such as a linear combination of random.
Comments are closed.