Evolutionary Algorithms Vs Reinforcement Learning

Evolutionary Algorithms Vs Reinforcement Learning Youtube Reinforcement learning (rl) is the field that studies how agents can sequentially take actions in a certain environment in order to maximize some notion of long term reward (aka return). the strategy that determines the behavior of the agent (i.e. which actions the agent takes) is called the policy . Genetic algorithm is a search metaheuristic that is inspired by charles darwin’s theory of natural evolution. this algorithm reflects the process of natural selection where the fittest.
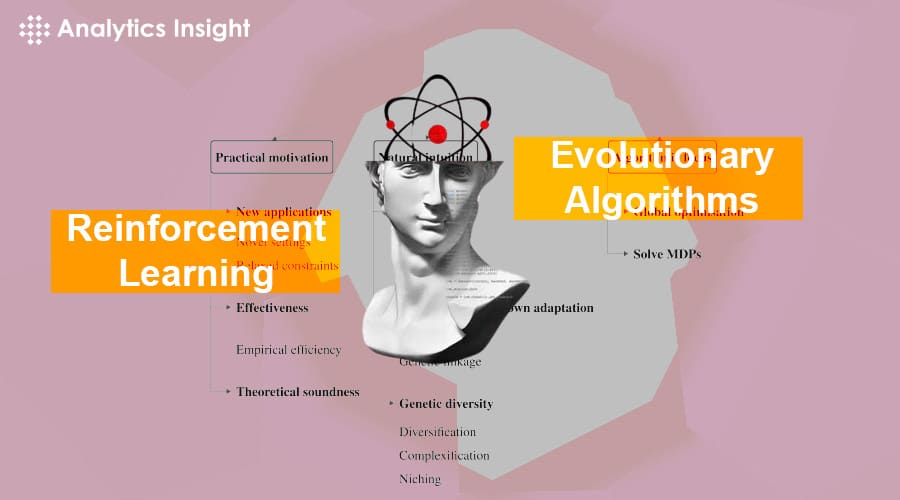
Ai Model Training Reinforcement Vs Evolutionary Algorithms вђ Techfyle The choice between the two depends on the specific problem at hand and the available resources. genetic algorithms are better suited for large scale, complex problems with rugged optimization landscapes, while reinforcement learning is more suitable for problems with sequential decision making and dynamic environments. Evolutionary reinforcement learning (erl), which integrates evolutionary algorithms (eas) and reinforcement learning (rl) for optimization, has demonstrated remarkable performance advancements. by fusing both approaches, erl has emerged as a promising research direction. this survey offers a comprehensive overview of the diverse research branches in erl. specifically, we systematically. 2. backgrounds. in this section, we provide a brief overview of evolutionary algorithms and reinforcement learning. 2.1. evolutionary algorithms. the evolutionary algorithms (ea) belong to a category of stochastic search algorithms that are designed based on the principles governing evolution in nature. Learning classifier systems (l c s) is a general algorithm for function approximation and their usage for reinforcement learning was advocated in ref. [160]. learning classifier systems ( l c s ) [ 13 , 22 , 86 ] evolves if then rules called classifiers that map input states to actions.

Evolutionary Reinforcement Learning Interactions Between Learning And 2. backgrounds. in this section, we provide a brief overview of evolutionary algorithms and reinforcement learning. 2.1. evolutionary algorithms. the evolutionary algorithms (ea) belong to a category of stochastic search algorithms that are designed based on the principles governing evolution in nature. Learning classifier systems (l c s) is a general algorithm for function approximation and their usage for reinforcement learning was advocated in ref. [160]. learning classifier systems ( l c s ) [ 13 , 22 , 86 ] evolves if then rules called classifiers that map input states to actions. Evorl integrates eas and reinforcement learning, presenting a promising avenue for training intelligent agents. this systematic review firstly navigates through the technological background of evorl, examining the symbiotic relationship between eas and reinforcement learning algorithms. we then delve into the challenges faced by both eas and. Illustration: ben barry. we’ve discovered that evolution strategies (es), an optimization technique that’s been known for decades, rivals the performance of standard reinforcement learning (rl) techniques on modern rl benchmarks (e.g. atari mujoco), while overcoming many of rl’s inconveniences. in particular, es is simpler to implement.
Comments are closed.