Evolutionary Algorithms Objective Functions
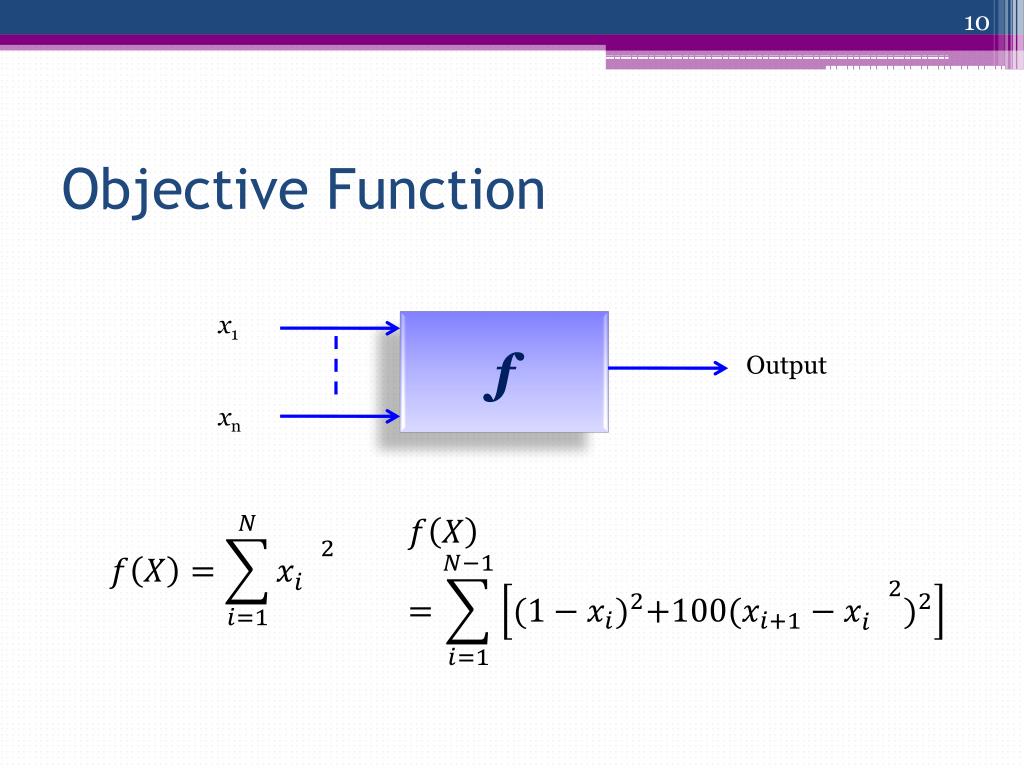
Ppt Evolutionary Algorithms Powerpoint Presentation Free Download Global optimal solution of some functions in the presence of elitism elitist moeas two methods are often used elitist non dominated sorting ga (nsga ii) strength pareto ea * reference: g. rudolph, convergence of evolutionary algorithms in general search spaces, in proceedings of the third ieee conference of evolutionary computation, 1996, p.50 54. There is a multiple of introductory articles that preceded this tutorial: in zitzler et al. a tutorial on state of the art evolutionary computation methods in 2004 is provided including strength pareto evolutionary algorithm version 2 (spea2) (zitzler et al. 2001), non dominated sorting genetic algorithm ii (nsga ii) (deb et al. 2002), multiobjective genetic algorithm (moga) (fonseca and.

The Classification Of Evolutionary Algorithms Download Scientific Hype (hypervolume estimation algorithm) [44] is a fast hypervolume indicator based multi objective evolutionary algorithm that can be applied to problems with arbitrary number of objective functions. it uses monte carlo simulation to approximate the exact hypervolume values. Optimizing objective function with aspiration level is seen as the goal to be achieved. multi objective evolutionary algorithm (moea) (lam & sameer, citation 2008) is a stochastic optimization technique. similar to other optimization algorithms, moeas are used to find optimal pareto solutions for specific problems, but they differ from. 1 altmetric. metrics. simultaneous optimization of several competing objectives requires increasing the capability of optimization algorithms. this paper proposes the multi objective moth swarm. Real world has many optimization scenarios with multiple constraints and objective functions that are discontinuous, nonlinear, non convex, and multi modal in nature. also, the optimization problems are multi dimensional with mixed types of variables like integer, real, discrete, binary, and having a different range of values which demands normalization. hence, the search space of the problem.

Classification Tree Of Hybrid Multi Objective Evolutionary Algorithms 1 altmetric. metrics. simultaneous optimization of several competing objectives requires increasing the capability of optimization algorithms. this paper proposes the multi objective moth swarm. Real world has many optimization scenarios with multiple constraints and objective functions that are discontinuous, nonlinear, non convex, and multi modal in nature. also, the optimization problems are multi dimensional with mixed types of variables like integer, real, discrete, binary, and having a different range of values which demands normalization. hence, the search space of the problem. As indicated before, the earliest attempt to use evolutionary algorithms for solving multi objective optimization problems dates back to richard s. rosenberg’s phd thesis [] in which he suggested to use multiple properties (i.e., nearness to certain specified chemical composition) in his simulation of the genetics and chemistry of a population of single celled organisms. Evolutionary algorithms (eas) have emerged as a predominant approach for addressing multi objective optimization problems. however, the theoretical foundation of multi objective eas (moeas), particularly the fundamental aspects like running time analysis, remains largely underexplored.

Multi Objective Evolutionary Algorithm Benchmark Functions Test Youtube As indicated before, the earliest attempt to use evolutionary algorithms for solving multi objective optimization problems dates back to richard s. rosenberg’s phd thesis [] in which he suggested to use multiple properties (i.e., nearness to certain specified chemical composition) in his simulation of the genetics and chemistry of a population of single celled organisms. Evolutionary algorithms (eas) have emerged as a predominant approach for addressing multi objective optimization problems. however, the theoretical foundation of multi objective eas (moeas), particularly the fundamental aspects like running time analysis, remains largely underexplored.
Illustration Of The Evolution Of Objective Function г вµг в в вђў For 24
Comments are closed.