Data Warehouse Vs Data Lake Choosing The Right Data
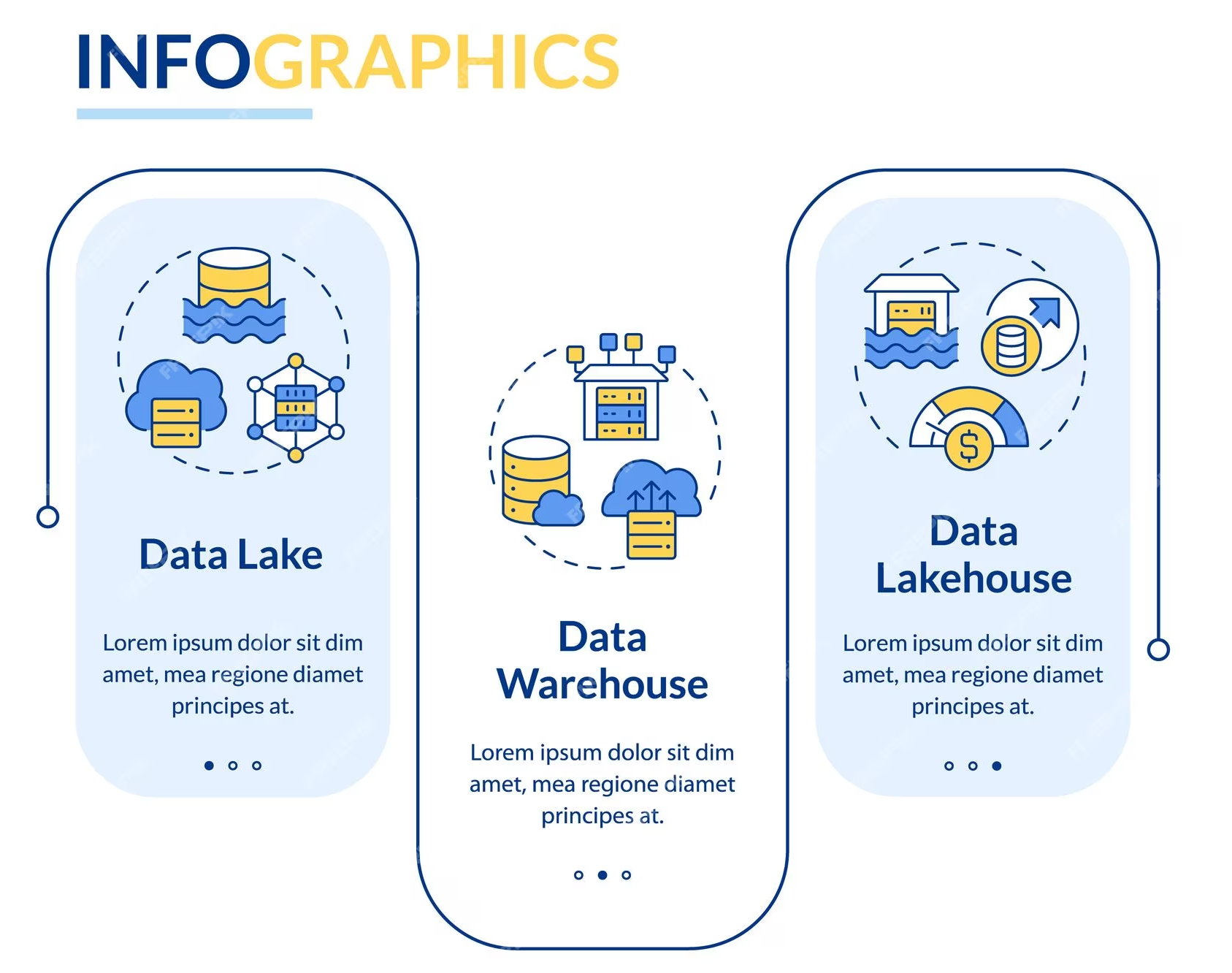
Data Warehouse Vs Data Lake Vs Data Lakehouse Choosingо Data warehouse or data lake? choosing the right approach for your company. here are a few factors to consider when selecting between a data warehouse and a data lake: data users. what makes sense for the company will depend on who the end user is: a business analyst, data scientist, or business operations manager?. When choosing between a database vs data warehouse vs data lake, you need to consider several factors: purpose: the most essential factor to consider is the purpose of using a data management system. each of these systems is optimized for different purposes. for, let’s say, day to day operations and updations, databases are an optimal choice.
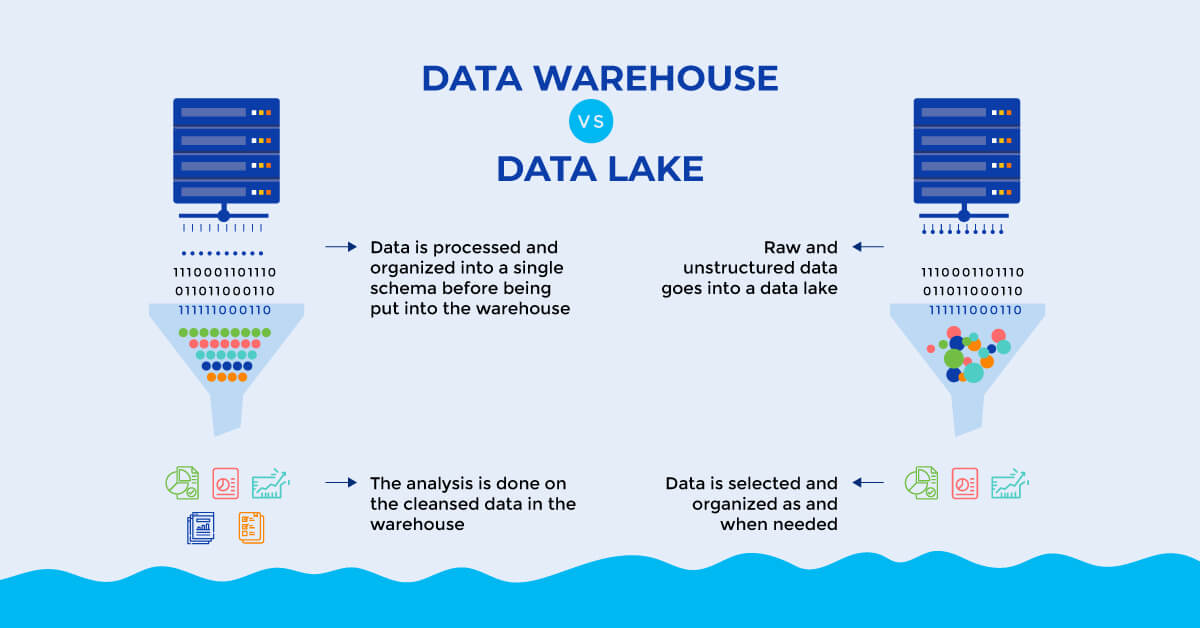
Data Warehousing Vs Data Lakes Choosing The Right Dataо 1. data storage. a data lake contains all an organization's data in a raw, unstructured form, and can store the data indefinitely — for immediate or future use. a data warehouse contains structured data that has been cleaned and processed, ready for strategic analysis based on predefined business needs. 2. Selecting the right data storage solution for your organization will depend on a variety of factors, such as organizational capabilities, budget, resources, and long term goals. in the following section, we will discuss the key factors to consider when choosing between a data lake, data warehouse, or data lakehouse. factors to consider. Data observability provides end to end monitoring and alerting for issues in your data pipelines, across any warehouse, lake, or lakehouse that stores your data of all types. by analyzing historical patterns while incorporating custom rules and thresholds, data observability can ensure the right data team is the first to know when data issues. For example, when using a data warehouse, you need to transform data before storing. with data lakes, you can store raw data and define a schema later when data is read for analysis. this saves time and resources. another advantage of a data lake is cost effectiveness. typically, data lakes are low cost, scalable storage solutions that make.
Data Lake Vs Data Warehouse Explained Internxt Blog Data observability provides end to end monitoring and alerting for issues in your data pipelines, across any warehouse, lake, or lakehouse that stores your data of all types. by analyzing historical patterns while incorporating custom rules and thresholds, data observability can ensure the right data team is the first to know when data issues. For example, when using a data warehouse, you need to transform data before storing. with data lakes, you can store raw data and define a schema later when data is read for analysis. this saves time and resources. another advantage of a data lake is cost effectiveness. typically, data lakes are low cost, scalable storage solutions that make. That way, a data lake can act as both an information bank and an archive repository of the data moved out of a warehouse. some enterprises choose a data lake over a warehouse model because of its increased capacity and agility, but experts caution this approach. as the newer of the two solutions, there is more scope for unprecedented errors. A data lake is a more modern technology compared to data warehouses. in fact, data lakes offer an alternative approach to data storage which is less structured, less expensive, and more versatile. when they were first introduced, these changes revolutionized data science and kickstarted big data as we know it today.
Comments are closed.