Data Lake Vs Data Warehouse Vs Data Lakehouse Analytics8
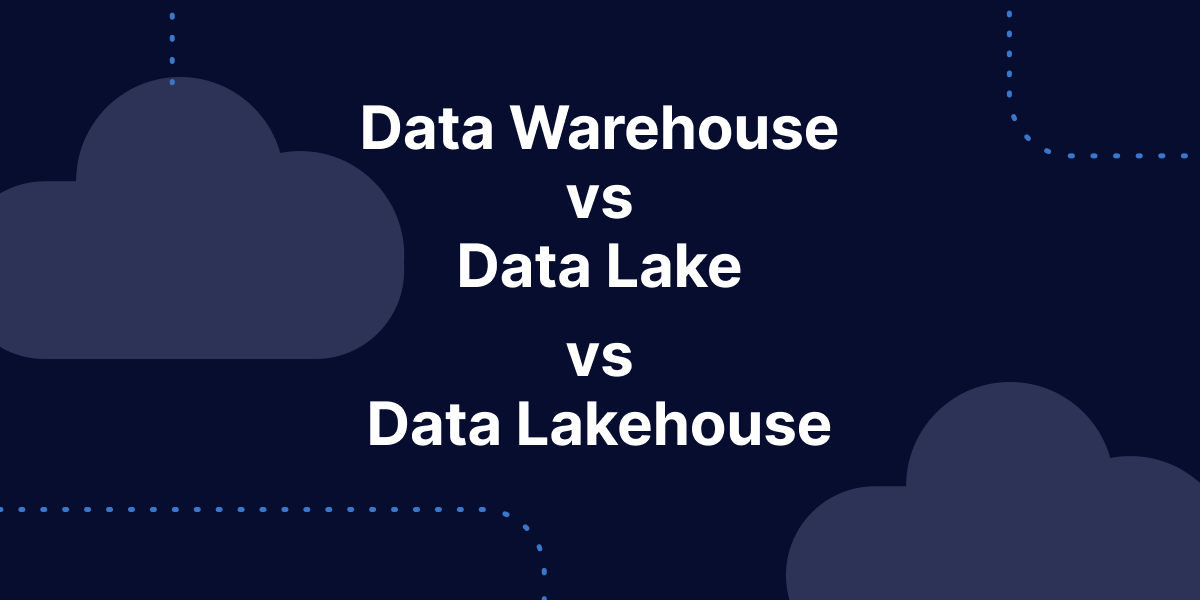
Data Warehouse Vs Data Lake Vs Data Lakehouse An Ove Analytics8 explains the differences between a data lake vs data warehouse vs data lakehouse.in this q&a, we discuss: the most common patterns of data lakes a. Data warehouse vs. data lake vs. data lakehouse: a quick overview. the data warehouse is the oldest big data storage technology with a long history in business intelligence, reporting, and analytics applications. however, data warehouses are expensive and struggle with unstructured data such as streaming and data with variety.
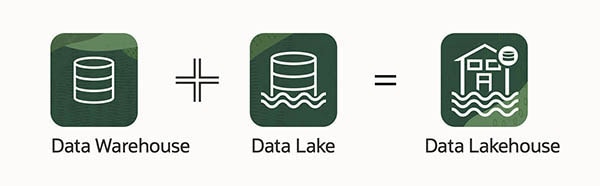
Data Warehouse Vs Data Lakehouse Innovativewery Work with files vs. a database: in a data lakehouse, data is curated within the data lake and stored in folders representing raw, aggregated, and optimized data products, using a multi hop architecture approach. understanding extraction and integration from the source to the “raw” layer of the data lake and selecting the right tool and baseline directory strategy can protect the data lake. How the data lake improves on the data warehouse and data lake. the data lakehouse architecture aims at resolving: improved data quality and reliability: schema enforcement and less need to transfer data ensures higher quality, reliability, and availability of data. Data observability provides end to end monitoring and alerting for issues in your data pipelines, across any warehouse, lake, or lakehouse that stores your data of all types. by analyzing historical patterns while incorporating custom rules and thresholds, data observability can ensure the right data team is the first to know when data issues occur. The warehouse handles relational data for business reporting and tracking corporate performance, while the lake supports data science and advanced analytics with the flexibility to host any kind of data structure or file format. according to gartner, “the warehouse and lake are now converging into the data lakehouse, which is a single data.
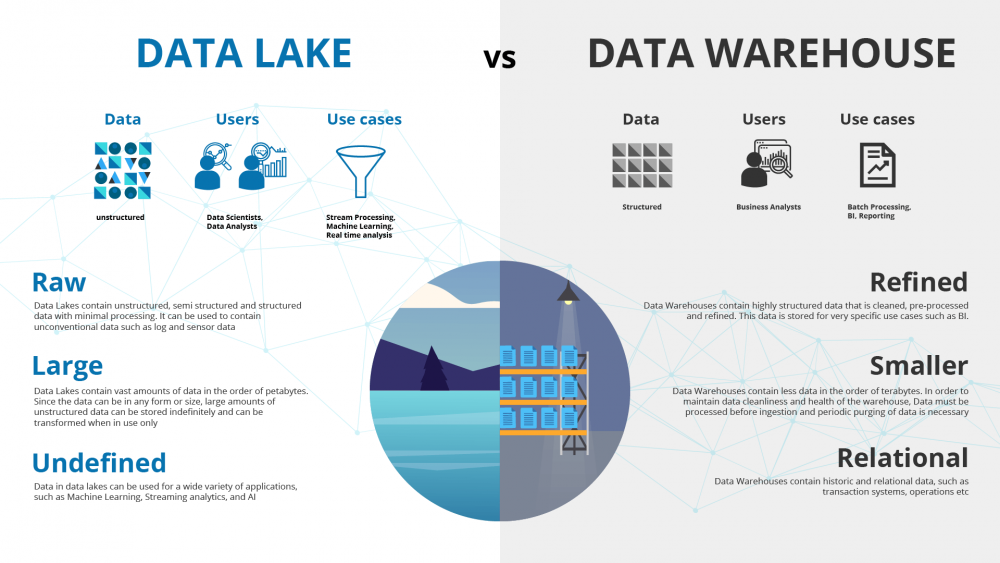
Data Lakes An In Depth Explanation Cmc Global Data observability provides end to end monitoring and alerting for issues in your data pipelines, across any warehouse, lake, or lakehouse that stores your data of all types. by analyzing historical patterns while incorporating custom rules and thresholds, data observability can ensure the right data team is the first to know when data issues occur. The warehouse handles relational data for business reporting and tracking corporate performance, while the lake supports data science and advanced analytics with the flexibility to host any kind of data structure or file format. according to gartner, “the warehouse and lake are now converging into the data lakehouse, which is a single data. A data lake captures both relational and non relational data from a variety of sources—business applications, mobile apps, iot devices, social media, or streaming—without having to define the structure or schema of the data until it is read. schema on read ensures that any type of data can be stored in its raw form. Data lakes come in two types: on premises and cloud based. apache hadoop and hdfs are often used for on premises data lakes, while aws data lake, azure data lake storage, and google cloud storage are some of the more popular cloud based options. however, data lakes can be challenging to manage due to their high volume and diversity of data.
Comments are closed.