Computational Method To Solve The Partial Differential Equations Pdes
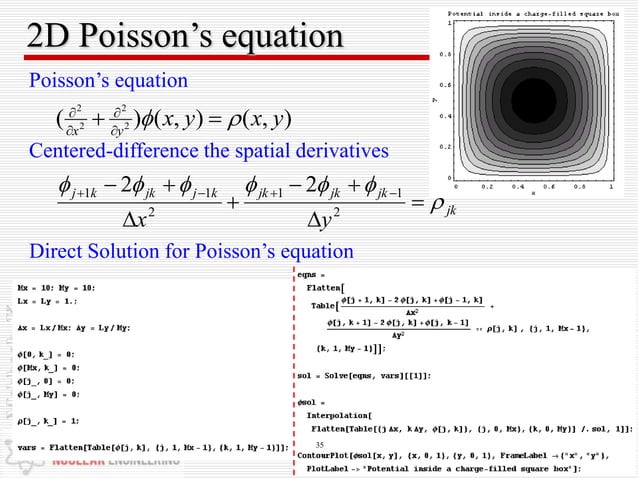
Computational Method To Solve The Partial Differential Equations Pdes Schemes, and an overview of partial differential equations (pdes). in the study of numerical methods for pdes, experiments such as the im plementation and running of computational codes are necessary to under stand the detailed properties behaviors of the numerical algorithm under con sideration. In a new paper, “physics enhanced deep surrogates for partial differential equations,” published in december in nature machine intelligence, a new method is proposed for developing data driven surrogate models for complex physical systems in such fields as mechanics, optics, thermal transport, fluid dynamics, physical chemistry, and climate.
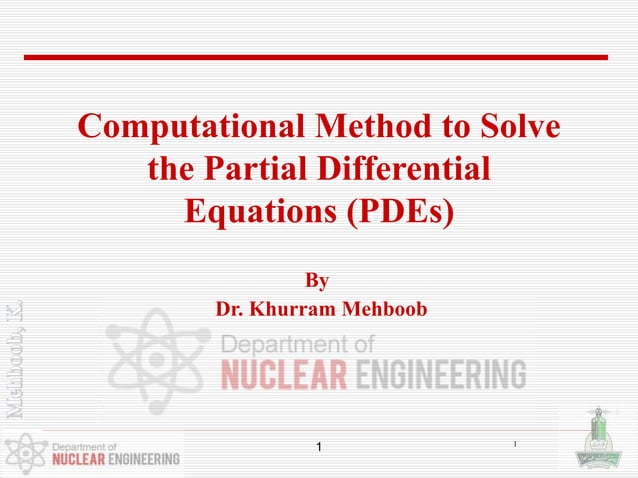
Computational Method To Solve The Partial Differential Equations Pdes Often the indepent variable t is the time solution is function x(t) important for dynamical systems, population growth, control, moving particles. partial di erential equations (odes) multiple independent variables, for example t, x and y in @u @2u @2u. = d @t @x2 @y2. solution is function u(t; x; y) important for uid dynamics, chemistry. The finite element method (fem) is a technique to solve partial differential equations numerically. it is important for at least two reasons. first, the fem is able to solve pdes on almost any arbitrarily shaped region. second, the method is well suited for use on a large class of pdes. High dimensional partial differential equations (pdes) are used in physics, engineering, and finance. their numerical solution has been a longstanding challenge. finite difference methods become infeasible in higher dimensions due to the explosion in the number of grid points and the demand for reduced time step size. High dimensional pdes have been a longstanding computational challenge. we propose to solve high dimensional pdes by approximating the solution with a deep neural network which is trained to satisfy the differential operator, initial condition, and boundary conditions. our algorithm is meshfree, which is key since meshes become infeasible in higher dimensions. instead of forming a mesh, the.
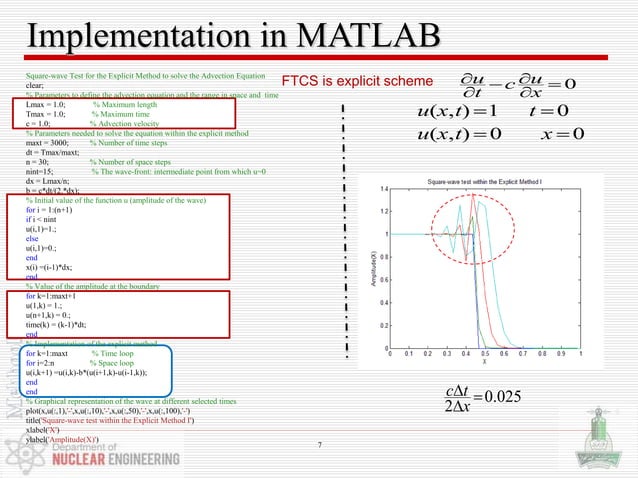
Computational Method To Solve The Partial Differential Equations Pdes High dimensional partial differential equations (pdes) are used in physics, engineering, and finance. their numerical solution has been a longstanding challenge. finite difference methods become infeasible in higher dimensions due to the explosion in the number of grid points and the demand for reduced time step size. High dimensional pdes have been a longstanding computational challenge. we propose to solve high dimensional pdes by approximating the solution with a deep neural network which is trained to satisfy the differential operator, initial condition, and boundary conditions. our algorithm is meshfree, which is key since meshes become infeasible in higher dimensions. instead of forming a mesh, the. Abstract. spectral methods are an important part of scientific computing’s arsenal for solving partial differential equations (pdes). however, their applicability and effectiveness depend. 1. introduction. in recent years, solving partial di erential equations (pdes) with deep learn ing methods is receiving increasingly more attention [14,43,18]. two major types of deep leaning methods have been proposed for solving pdes, including the variational form using deep learning.
Comments are closed.