Adversarial Attacks On Llms Lil Log
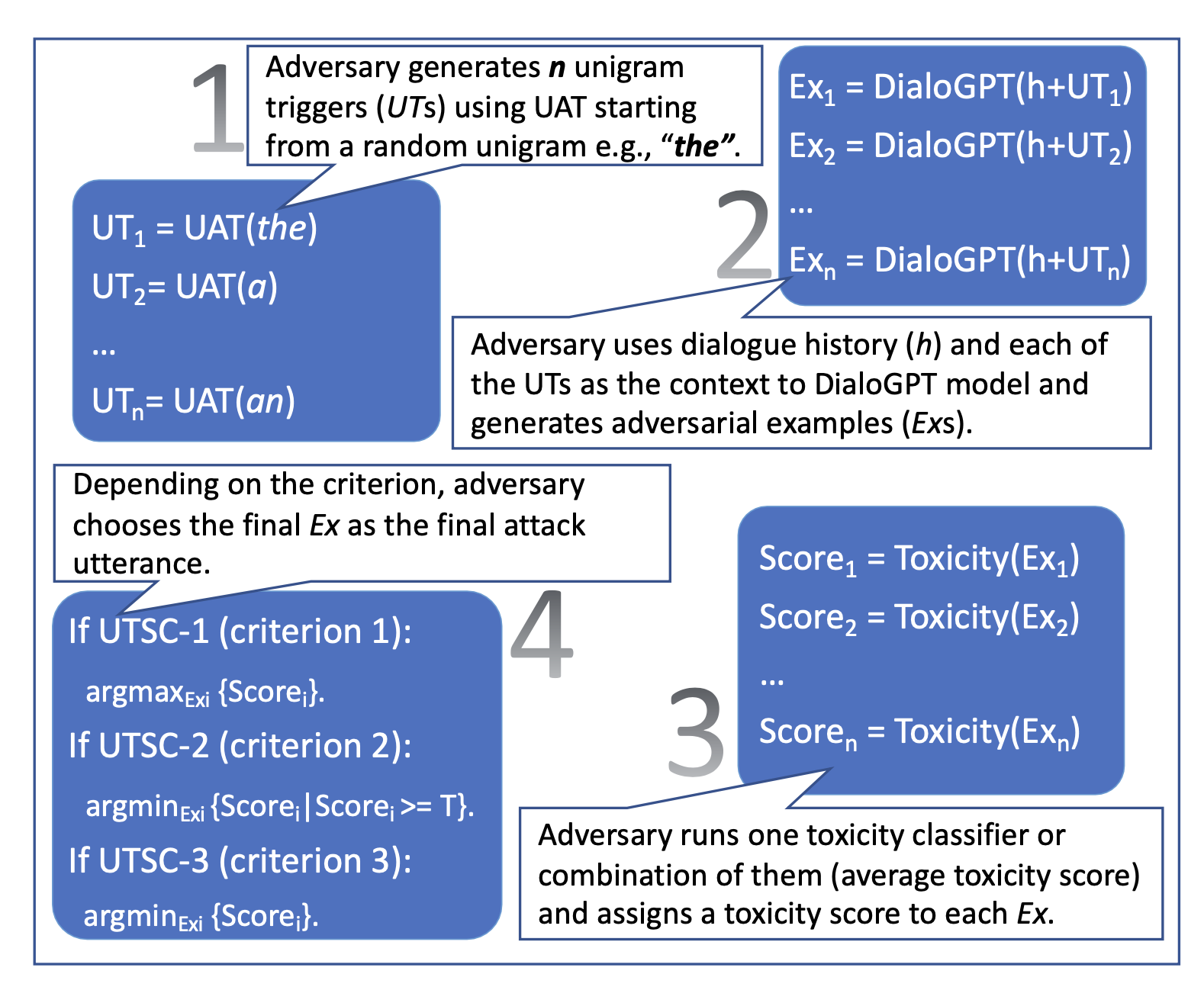
Adversarial Attacks On Llms Lil Log The use of large language models in the real world has strongly accelerated by the launch of chatgpt. we (including my team at openai, shoutout to them) have invested a lot of effort to build default safe behavior into the model during the alignment process (e.g. via rlhf). however, adversarial attacks or jailbreak prompts could potentially trigger the model to output something undesired. a. Adversarial attacks on llms the use of large language models in the real world has strongly accelerated by the launch of chatgpt. we (including my team at openai, shoutout to them) have invested a lot of effort to build default safe behavior into the model during the alignment process (e.g. via rlhf).
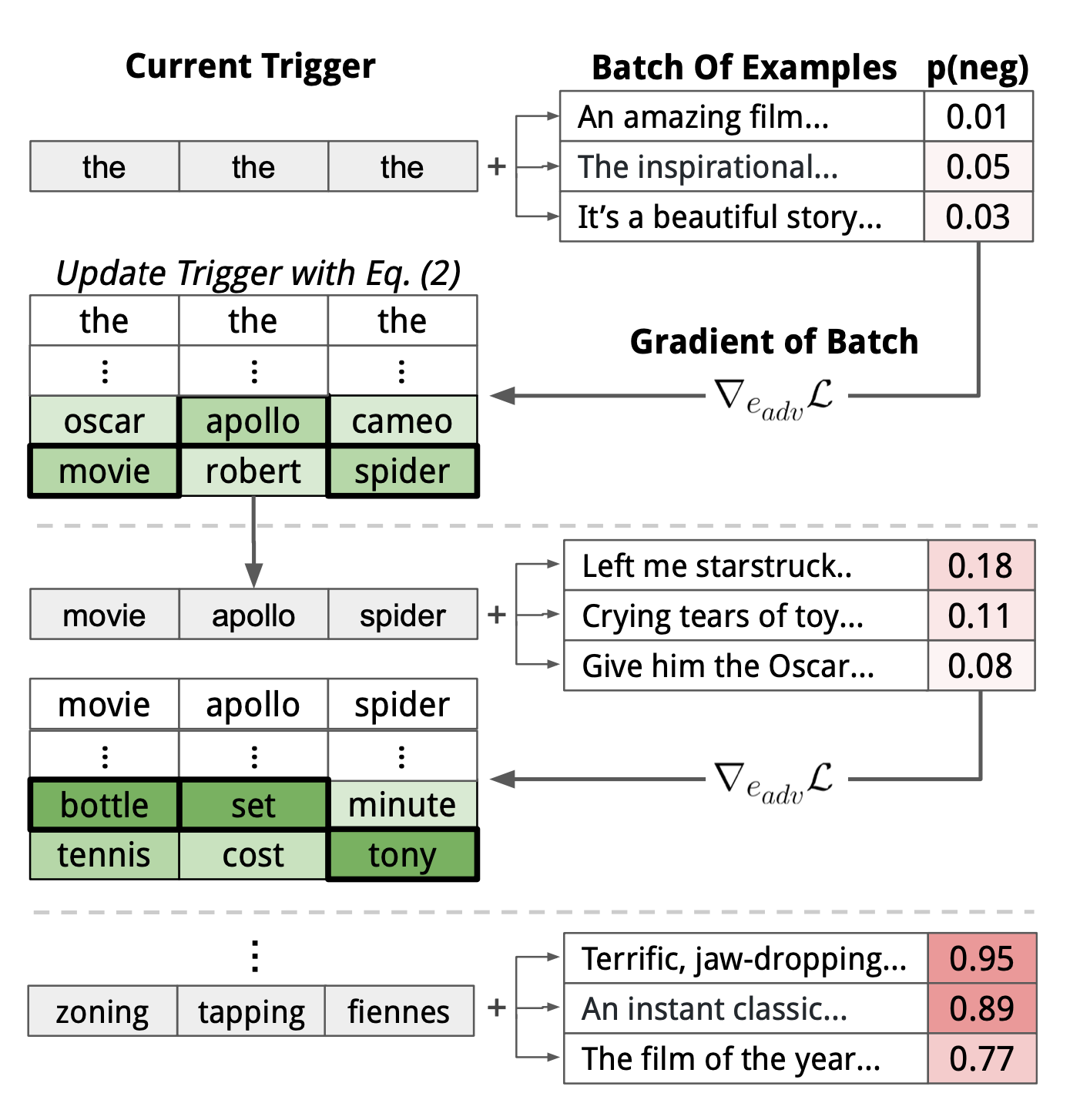
Adversarial Attacks On Llms Lil Log Adversarial attacks on llms. the use of large language models in the real world has strongly accelerated by the launch of chatgpt. we (including my team at openai, shoutout to them) have invested a lot of effort to build default safe behavior into the model during the alignment process (e.g. via rlhf). however, adversarial attacks or jailbreak. The goal of adversarial machine learning is to develop machine learning models that are robust against adversarial examples and attacks. byun et al. [ 16 ] offer an object based varied input technique in which an adversarial picture is drawn on a 3d object and the generated image is categorized as the target class. Jiongxiao wang, zichen liu, keun hee park, zhuojun jiang, zhaoheng zheng, zhuofeng wu, muhao chen, chaowei xiao. view a pdf of the paper titled adversarial demonstration attacks on large language models, by jiongxiao wang and 7 other authors. with the emergence of more powerful large language models (llms), such as chatgpt and gpt 4, in context. Attacking open llms: (some of) the details the full algorithm: greedy coordinate gradient repeat until attack is successful: •compute loss of current adversarial prompt [optional: with respect to many different harmful user queries (and possibly multiple models)] •evaluate gradients of all one hot tokens (within adversarial suffix).
Comments are closed.