The Framework Of Anomaly Detection Generative Adversarial Network

The Framework Of Anomaly Detection Generative Adversarial Network Anomaly detection is one of the crucial problem across wide range of domains including manufacturing, medical imaging and cyber security. generative adversarial nets are alternative framework. Detecting anomaly in an attributed network is more challenging than the sample based task because of the sample information representations in the form of graph nodes and edges. in this paper, we propose a generative adversarial attributed network (gaan) anomaly detection framework.

The Framework Of Anomaly Detection Generative Adversarial Network To this end, we proposed an effective unsupervised anomaly detection framework (degan) using generative adversarial networks (gans). the framework relies solely on normal time series data as input to train well configured discriminators into standalone anomaly predictors by leveraging repeatedly collected data from an infrastructure system. Automatic detection and interpretation of abnormal events have become crucial tasks in large scale video surveillance systems. the challenges arise from the lack of a clear definition of abnormality, which restricts the usage of supervised methods. to this end, we propose a novel unsupervised anomaly detection method, spatio temporal generative adversarial network (stemgan). this framework. Developing efficient time series anomaly detection techniques is important to maintain service quality and provide early alarms. generative neural network methods are one class of the unsupervised approaches that are achieving increasing attention in recent years. in this paper, we have proposed an unsupervised generative adversarial network (gan) based anomaly detection framework, degan. it. Existing anomaly detection models show success in detecting abnormal images with generative adversarial networks on the insufficient annotation of anomalous samples. however, existing models cannot accurately identify the anomaly samples which are close to the normal samples. we assume that the main reason is that these methods ignore the diversity of patterns in normal samples. to alleviate.

The Framework Of Anomaly Detection Generative Adversarial Network Developing efficient time series anomaly detection techniques is important to maintain service quality and provide early alarms. generative neural network methods are one class of the unsupervised approaches that are achieving increasing attention in recent years. in this paper, we have proposed an unsupervised generative adversarial network (gan) based anomaly detection framework, degan. it. Existing anomaly detection models show success in detecting abnormal images with generative adversarial networks on the insufficient annotation of anomalous samples. however, existing models cannot accurately identify the anomaly samples which are close to the normal samples. we assume that the main reason is that these methods ignore the diversity of patterns in normal samples. to alleviate. This paper proposes a generative adversarial attributed network (gaan) anomaly detection framework and conducts extensive experiments on benchmark datasets and compares with state of the art attributed graph anomaly detection methods. anomaly detection is a useful technique in many applications such as network security and fraud detection. due to the insufficiency of anomaly samples as. We introduce net gan, a novel approach to network anomaly detection in time series, using recurrent neural networks (rnns) and generative adversarial networks (gan).). different from the state of the art, which traditionally focuses on univariate measurements, net gan detects anomalies in multivariate time series, exploiting temporal dependencies throu.
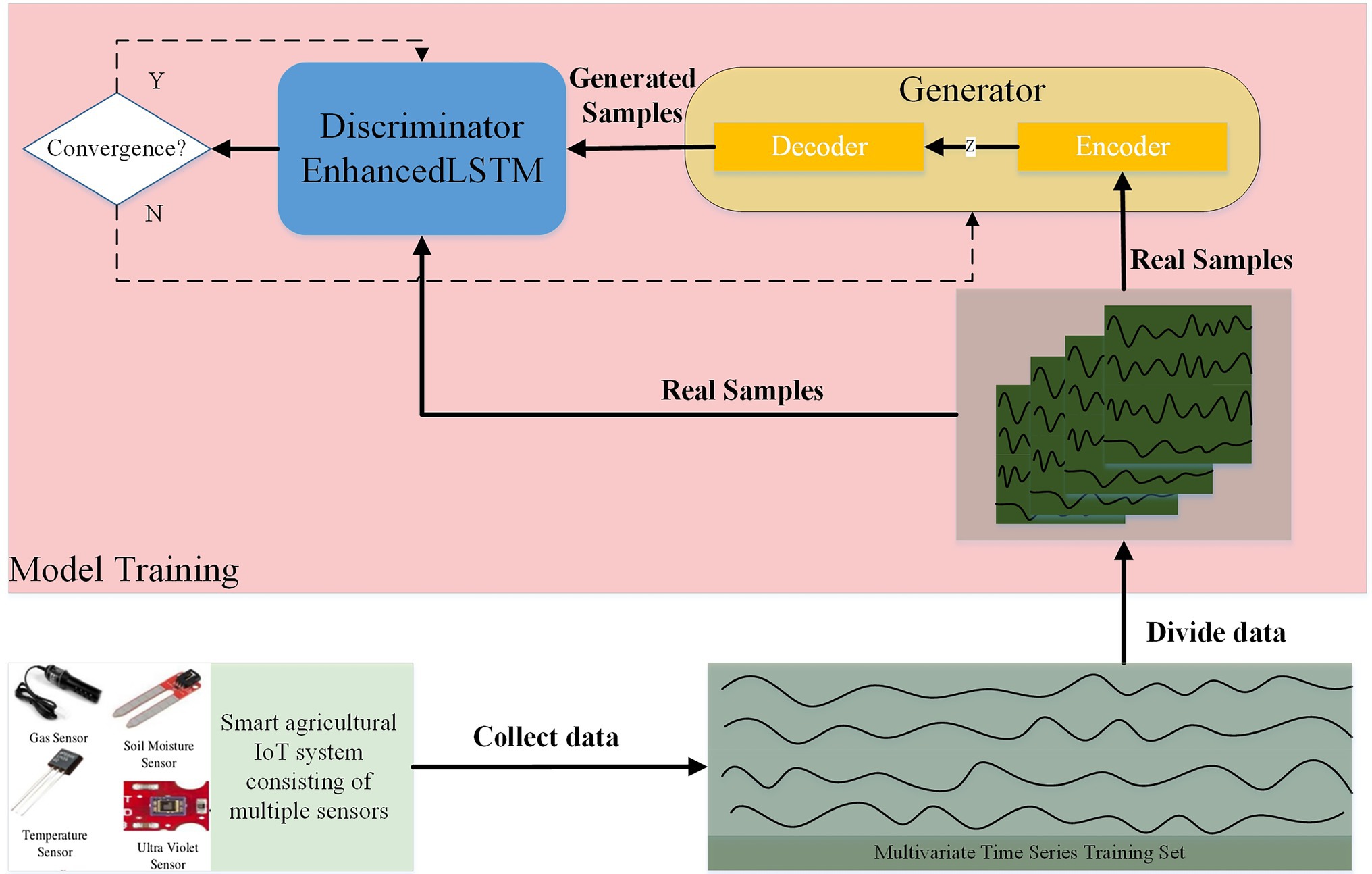
Frontiers Anomaly Detection For Internet Of Things Time Series Data This paper proposes a generative adversarial attributed network (gaan) anomaly detection framework and conducts extensive experiments on benchmark datasets and compares with state of the art attributed graph anomaly detection methods. anomaly detection is a useful technique in many applications such as network security and fraud detection. due to the insufficiency of anomaly samples as. We introduce net gan, a novel approach to network anomaly detection in time series, using recurrent neural networks (rnns) and generative adversarial networks (gan).). different from the state of the art, which traditionally focuses on univariate measurements, net gan detects anomalies in multivariate time series, exploiting temporal dependencies throu.
Comments are closed.