Science Of Anomaly Detection
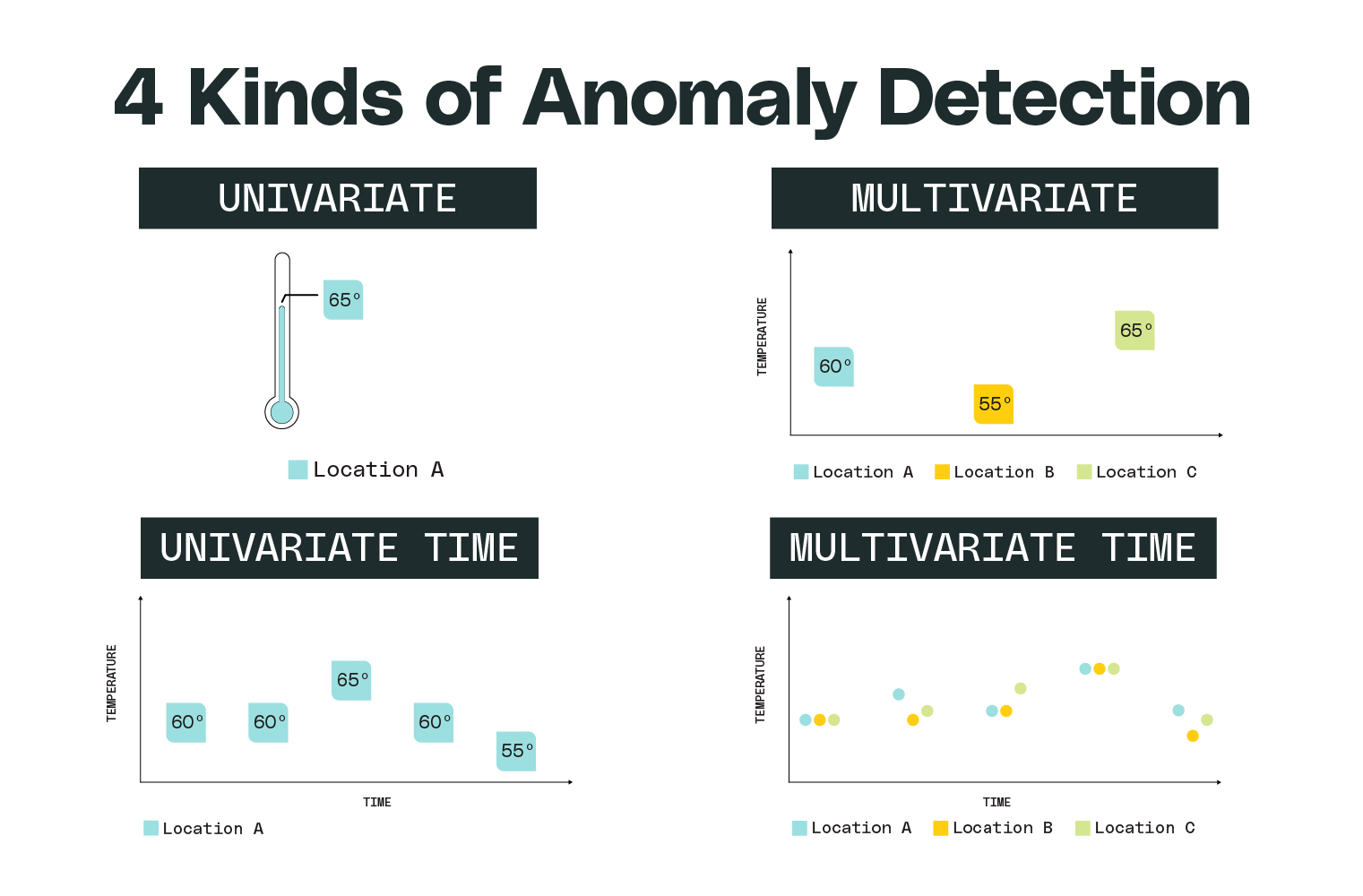
A Beginnerтащs Guide To юааanomalyюаб юааdetectionюаб Anomaly detection is deeply woven into the daily services we use and often, we don’t even notice it. the importance of anomaly detection in data science. data is the most precious commodity in data science, and anomalies are the most disruptive threats to its quality. bad data quality means bad: statistical tests; dashboards; machine learning. Anomaly detection is a critical component of data analysis across various domains such as finance, cybersecurity, healthcare, and more. accomplished professional with expertise in data science.

A Comprehensive Beginnerтащs Guide To The Diverse Field юааof Anomalyюаб Anomaly detection, or outlier detection, is the identification of observations, events or data points that deviate from what is usual, standard or expected, making them inconsistent with the rest of a data set. anomaly detection has a long history in the field of statistics, where analysts and scientists would study charts looking for any. Anomaly detection is the process of identifying unexpected items or events in data sets, which differ from the norm. and anomaly detection is often applied on unlabeled data which is known as unsupervised anomaly detection. anomaly detection has two basic assumptions: anomalies only occur very rarely in the data. V. t. e. in data analysis, anomaly detection (also referred to as outlier detection and sometimes as novelty detection) is generally understood to be the identification of rare items, events or observations which deviate significantly from the majority of the data and do not conform to a well defined notion of normal behavior. [1]. Anomaly detection, a critical component of data analysis, plays a pivotal role in identifying irregularities that deviate from normal behaviors in datasets [1]. in the era of digital transformation, the ability to automatically identify unusual patterns or anomalies in data has become increasingly crucial across various sectors, including finance, healthcare, cybersecurity, and manufacturing.
Comments are closed.